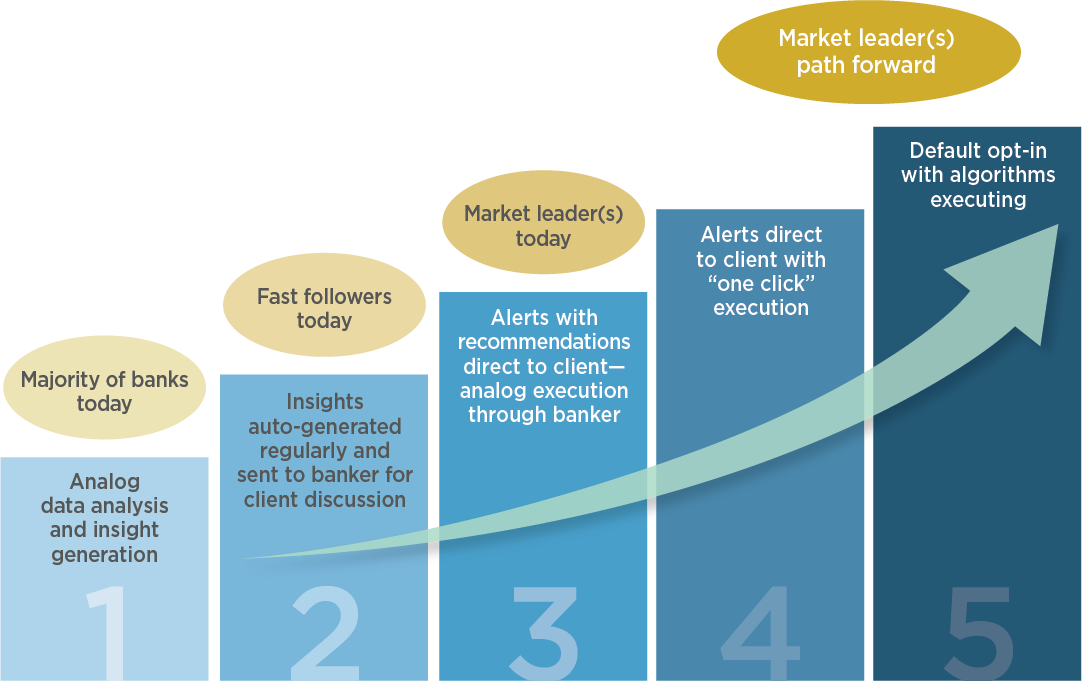
Rapid advances in data curation and technology are changing the measurements of success in the commercial payments business.
Looking ahead, analytics-driven recommendations to clients will be enabled by comprehensive data sets, including elements such as real-time payments telemetry data, risk/hedging data, and best-in-class efficiency information augmented by artificial intelligence (AI) and machine learning (ML).
These insights will be shared simultaneously with the company and bankers, thus improving the efficiency of idea flow to clients and prospects. The recommendations will be "persona-specific" to individual roles inside client organizations and based on their functional needs, company strategy and desired risk tolerance.
For example, a CFO at headquarters has different needs than an assistant treasurer at a subsidiary or regional treasury center.
Banks with the best recommendation engines—those powered by unique, highly curated data and superior predictive analytics—will separate themselves from the pack
The shift from episodic, banker-driven ideas to more continuous analytics-driven insights at the personal level will help clients optimize efficiency, risk and profitability.
For banks, separating the noise from value-added advice will be an important factor to gaining client trust in their recommendations. Incorporating ML on the back of client responses to insights will also help increase the value and precision of these recommendations.
Contextual insights will help the leaders gain market share and improve efficiency, as some elements of client-delivered value shift from man to machine.
The journey to differentiation requires a steadfast focus and continuous investment to turn episodic banker interactions to hyper-customized, value-added insights.
Download slides from a recent presentation I made. They cover: