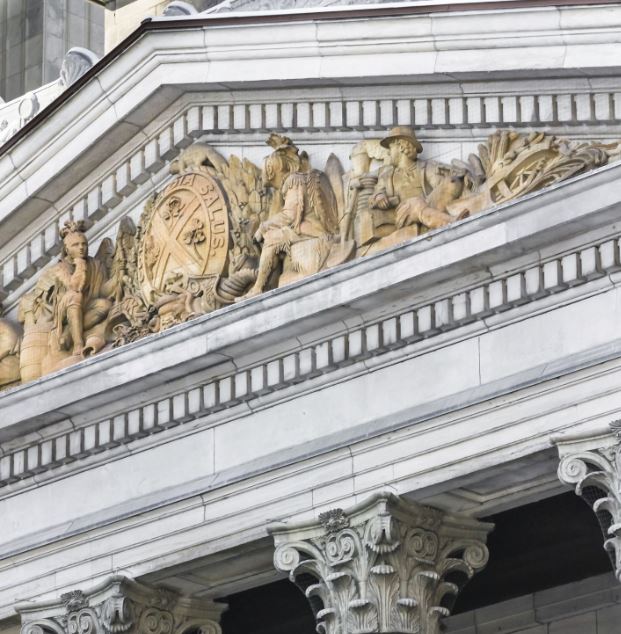
Not all markets need regulatory mandates for electronic trading to grow and thrive. Nearly 70% of volume traded in the U.S. Treasury market is done via e-trading platforms, with over 90% of the nearly $200 billion traded daily between market makers executed on the screen. The size and standard nature of the market, coupled in recent years with the relatively low profit margins for traditional dealers, have allowed increased automation to flood the market, benefiting institutional investors around the world.
Taking derivatives markets into account, automation levels are even higher. Nearly all of the U.S. interest-rate futures market is traded electronically, and Greenwich Associates research shows that over 60% of interest-rate swaps are traded via swap execution facilities.
The cash and derivative markets have become increasingly linked in recent years, with the tail sometimes wagging the dog. Futures volumes have occasionally topped volumes in the underlying bond market, and many market participants determine Treasury prices based on the futures market rather than the other way around.
What is lacking here, however, is a higher level of automation for investors trading U.S. Treasurys. While over 50% of volume is executed electronically today, the vast majority of that is via point-and-click RFQ. This has served the buy side well for years, allowing them to transfer hundreds of millions of dollars in bonds quickly and at what they perceive as good prices.
In the last two years, the RFQ process has received an automation overhaul, with RFQs for smaller orders being sent automatically to an algorithmically selected group of dealers. Many of those dealers respond with no human intervention, and the best price is then chosen automatically by the requesting machine.
But automation can go further. Providing the buy side with execution algos that can aggregate disparate liquidity pools, including order books and direct streams, is a must—and a complement to the tried and tested tools they have today.
The buy side executes thirty-three percent of U.S. equities volume and 10% of FX volume via algos today. Yet, while the dealer-to-dealer market for U.S. Treasurys is traded almost completely via trading algos and some large asset managers are evaluating their use, almost no trading between investors and dealers is algorithmically executed today.
The algos used by banks and market makers differ greatly from those needed by investors. And those used in equities cannot be cut and pasted into the bond market. Nevertheless, a return to market volatility and pressure on buy-side management fees are creating huge incentives to implement automation wherever possible—including algorithmic execution. But to make this possible, a few requirements must first be addressed.
Algorithms can’t make decisions without data as an input. While current U.S. Treasury prices are widely disseminated, trade- and tick-level data is harder to come by. Reporting requirements put into place last year produce data that is only consumed by regulators. While these rules are expected to change eventually and require more public reporting, more data is needed.
The top trading platforms possess information on not only every trade executed, but every price posted and every RFQ made. Utilizing this data to inform future trading decisions is a smart step. This trading information and similar historical data can also serve as the fuel for “machine learning” algorithms that get smarter the more they are used.
Futures market data can also fill in some of the information gaps, as trading activity between the futures and cash markets are highly correlated. Nevertheless, a view of the entire market remains elusive and difficult to cobble together.
Analytics that allow traders to analyze both pre- and post-trade trading data are also key. Determining the benefits of trading algorithmically requires a way to measure the results. While transaction cost analysis (TCA) adoption is growing in fixed-income markets, it has yet to hit the mainstream, as it has in equities.
Algorithms and order book/aggregated stream trading are a bit like the chicken and the egg. Access to one would encourage more use of the other. For instance, increased access to trading via order books and direct streams for the buy side would further encourage experimentation with execution algos. The ability to continuously scan real-time prices across several markets without signaling trading intention make algos both possible and effective.
The buy side continues to show only limited interest in anonymous order-book trading today, despite the potential reduction in information leakage. They remain happy with the liquidity they receive via RFQ and the phone. Their interest in aggregating and trading via direct pricing streams is accelerating, however. LiquidityEdge’s market share growth over the past two quarters provides some evidence of that, for instance.
Early adopters in the equity market had been forced to build everything in-house—an expensive and slow proposition. But the U.S. Treasury market is in a better position today, able to quickly adopt new tools as they are developed and delivered by specialist providers. Trying before buying is therefore possible, and real-world results are always more convincing than marketing materials.
Electronic trading eventually leads to algorithmic trading. We’ve seen this in equities, futures and FX thus far. Dealers and market makers are early adopters of both electronic and algorithmic trading, with those tools eventually reaching the buy side. Again, we have seen this progression in equities, futures and FX. The U.S. Treasury market is next on both counts.
The fixed-income market has seen a huge surge of new data since the financial crisis, including everything from reported swaps trades to TRACE for Treasurys to continuous evaluated pricing. More data has led to more e-trading, which generates only more data. Implementing TCA to analyze that data will encourage even more e-trading and, soon, algorithmic trading by the buy side.
These changes will not occur overnight, nor will they happen all at once. Investors and traders are risk-takers in the market but are generally risk averse when it comes to new technology. While machine learning algos exist today for trading in the interest-rate market, the technology still needs to earn the trust of the trading desk—something that only comes with time and consistent positive results.